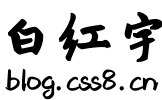
本文共 4918 字,大约阅读时间需要 16 分钟。
Overcoming Genetic Drop-outs in Variants-based Lineage Tracing from Single-cell RNA Sequencing Data
Understanding the Scope of Lineage Tracing Techniques
Lineage tracing is a critical approach in studying cellular differentiation, development, and cancer biology. There are primarily two categories of methods: intrusive and non-intrusive techniques. The former involves genetic modifications such as insertions or deletions, while the latter leverages high-throughput and non-invasive approaches like single-cell RNA sequencing (scRNA-seq).Among non-intrusive techniques, single-cell RNA sequencing has emerged as a powerful tool for lineage tracing. It identifies lineage changes by analyzing gene expression gradients, assuming temporal dynamics, or detecting genetic variants like chromosomal (chrM) and non-chrM mutations. ChrM mutations, characterized by mutations on the mitochondrial DNA, are more stable across cells, making them suitable for cross-individual lineage tracing. In contrast, non-chrM mutations, involving nuclear DNA regions not linked to the mitochondrial genome, vary more frequently, making them ideal for studying lineage dynamics within individual cells.
The Impact of Genetic Drop-outs
In scRNA-seq data, genetic drop-outs significantly affect lineage tracing. These drop-outs occur when certain genomic regions are not covered sufficiently, leading to missing data on specific alleles. For example, in heterozygous cells, only one of the two alleles may be detected, causing potential inaccuracies in lineage inference. Non-chrM variants are particularly prone to this issue, as their coverage is often lower than that of chrM mutations.The Advantages of Using SClineager for Lineage Tracing
To address the challenges posed by genetic drop-outs, we introduce SClineager, an innovative analysis framework designed specifically fornon-chrM variant-based lineage tracing. SClineager builds a hierarchical dependency model linking latent cell lineages to true genetic variants. By considering the coverage, allelic imbalance, and variant allele frequencies (VAFs), SClineager effectively mitigates drop-out effects.Initial Observations and Insights
Our analysis across multiple datasets, including those from tumor microenvironments, hematopoietic cells, and mouse models, revealed several key insights. The higher coverage of non-chrM variants provides a richer dataset for lineage tracing. However, unlike chrM mutations, non-chrM VAFs exhibit significant variability, necessitating careful handling to ensure accurate lineage reconstruction.Understanding Genetic Drop-outs in Depth
Genetic drop-outs primarily manifest in two forms:Operational Strategies for SClineager
We developed SClineager, which captures hierarchical relationships by considering variants with higher coverage and lower AAI. This approach ensures accurate lineage clustering, achieving over 99% accuracy. Cells within the same lineage tend to exhibit similar variants and VAFs.Applying SClineager Across Diverse Datasets
- Tumor Microenvironments: Our analysis of 969 CD45+ cells across five renal cell carcinoma patients revealed distinct clustering by VAFs.
- Hematopoietic Cells and TF1 Lineage: Primary hematopoietic cells and TF1 cells were neatly separated using SClineager-in inferred VAFs.
- Chronic Myeloid Leukemia (CML): SClineager uncovered novel genetic insights overlooked in standard gene expression analysis.
- Cutaneous T-cell Lymphoma (CTCL): Identified dominant TCR clones accurately separated from indigenous T cells, highlighting divergent sub-clones.
- Human Liver and Mouse Brain Mapping: High mutational load correlates with mid-zone gene modules in liver tissue, while mouse olfactory bulb variants showed strong anatomic correlations.
Practical Implementation Steps
Variant Calling
Use the QBRC mutation calling pipeline, aligning reads to the human reference genome with BWA-MEM. Employ tools like GATK, MuTect, VarScan, and Strelka2 for reliable variant detection.Data Filtering
Filter mutations with at least 7 reads in normal samples and 3 reads in tumor samples. Retain variants with allele frequencies significantly different from normal alleles.Implement SClineager
Apply our hierarchical clustering algorithm to group cells based on VAFs. SClineager reliably identifies lineages with high accuracy, ensuring robust downstream analyses.By employing SClineager, researchers can overcome genetic drop-outs and achieve more accurate lineage tracing, unlocking novel insights into cellular evolution and disease mechanisms.
发表评论
最新留言
关于作者
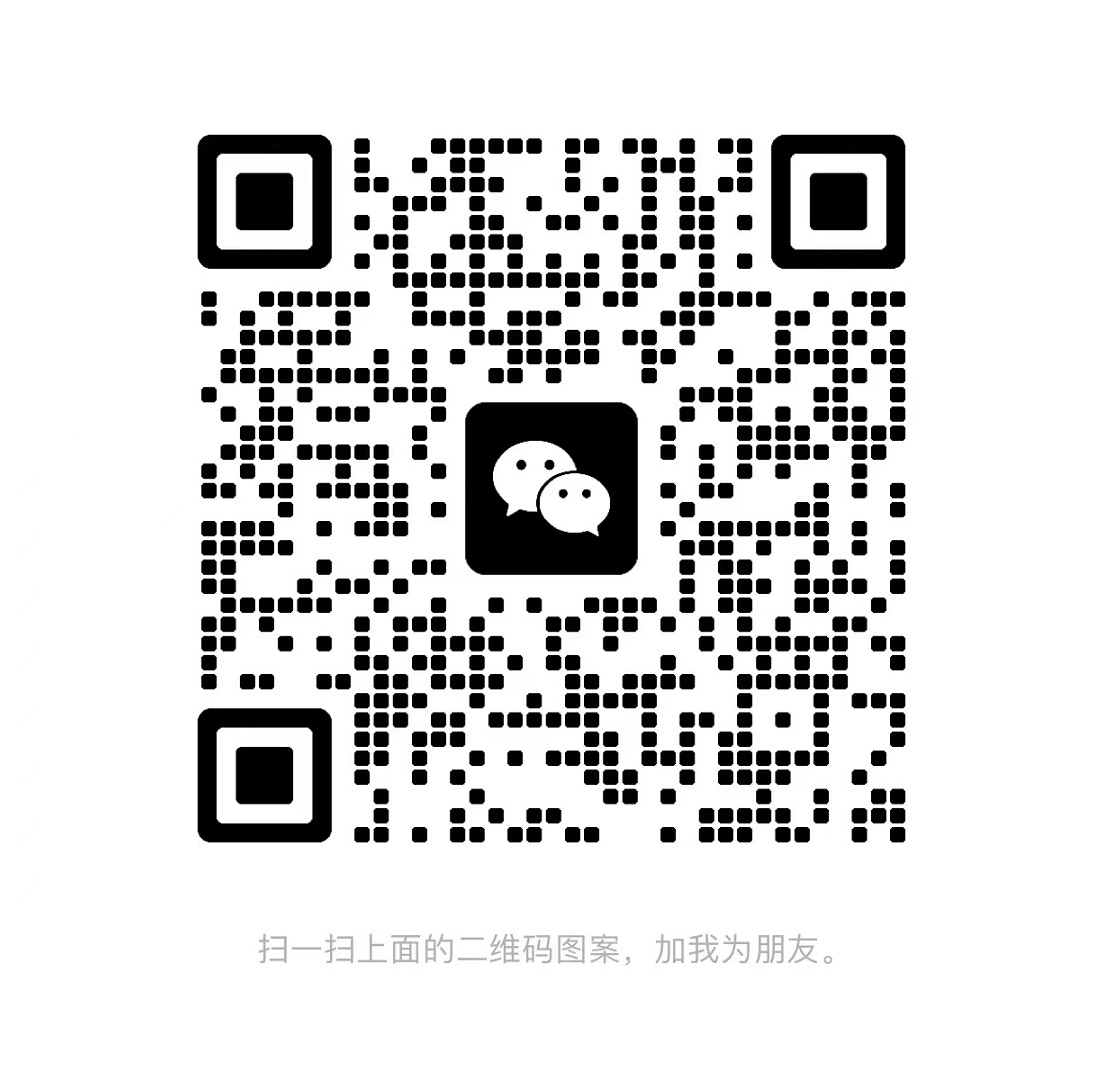