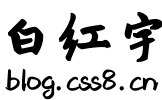
本文共 4927 字,大约阅读时间需要 16 分钟。
������TensorFlow���������������������������������
������������������������������������TensorFlow���������������������������������������������������������������������������������������������������������������
1. ������������
���������������������������������������������������
���������������������������������������������������������������������������
- ���������������������������������������������������
- ���������������������������������������������������ReLU������
- ������������������������������������������������������
- ���������������������������������������������������������
2. ������������
������������������������������������������������numpy���������������������������
x_data = np.linspace(-1, 1, 300, dtype=np.float32)x_data = np.reshape(x_data, (-1, 1)) # ���������������������������(300, 1)
������������������������������
noise = np.random.normal(0, 0.05, x_data.shape)y_data = np.square(x_data) - 0.5 + noise
���������
linspace
���������-1���1���300������������������������������������reshape
���������������������300���1������������������������������TensorFlow������������������������- ������
normal
������������������������������0���������������0.05��������������������������������������� y_data
���������������������������������0.5���������������������������������300������������
������������������TensorFlow������������
xs = tf.placeholder(tf.float32, [None, 1])ys = tf.placeholder(tf.float32, [None, 1])
3. ������������������
���������������������������������������������
# ���������hidden_layer = add_layer(xs, 1, 10, activation_function=tf.nn.relu)# ���������prediction = add_layer(hidden_layer, 10, 1, activation_function=None)
������add_layer
���������������
def add_layer(inputs, in_size, out_size, activation_function=None): # ��������������� weights = tf.Variable(tf.random_normal([in_size, out_size])) # ��������������� biases = tf.Variable(tf.zeros([1, out_size]) + 0.1) # ������������ wx_plus_b = tf.matmul(inputs, weights) + biases # ������������������ if activation_function is None: outputs = wx_plus_b else: outputs = activation_function(wx_plus_b) return outputs
���������
- ������������������������������������������������������������������������������������������
matmul
���������������������������������- ������������������������������������������������������������
- ������������������������������������������������������������
4. ������������
���������������������������������������
loss = tf.reduce_mean(tf.reduce_sum(tf.square(ys - prediction)))
���������
tf.square
���������������������������������������������tf.reduce_sum
������������������������������������������������������tf.reduce_mean
������������������������������������������������������
5. ������������
������������������������������������������������
train_step = tf.train.GradientDescentOptimizer(0.1).minimize(loss)
������������������
init = tf.global_variables_initializer()sess = tf.Session()sess.run(init)
���������������������
for i in range(1000): # ������ sess.run(train_step, feed_dict={xs: x_data, ys: y_data}) if i % 50 == 0: #��������� try: ax.lines.remove(lines[0]) except: pass prediction_value = sess.run(prediction, feed_dict={xs: x_data}) lines = ax.plot(x_data, prediction_value, 'r-', lw=5) plt.pause(0.5)
���������
GradientDescentOptimizer
������������������0.1���minimize
������������������������������������tf.Session
���������������������������������for
������������1000������- ���50������������������������������
6. ���������������
������matplotlib������������������������������������
fig = plt.figure()ax = fig.add_subplot(1,1,1)ax.scatter(x_data, y_data)plt.ion()plt.show()
7. ������
���������������������������������������������������������������������������������������������������������������������������������������������������������������������������������������������������������������������������������������������
8. ������������
������������������������������������������������������
- ���������������������������������������������������������
- ���������������������������������������L2������������
- ������������������������������������������Adam���
- ���������������������������������������������������������������������������������
������������������������������������������������������������������������������������������������
发表评论
最新留言
关于作者
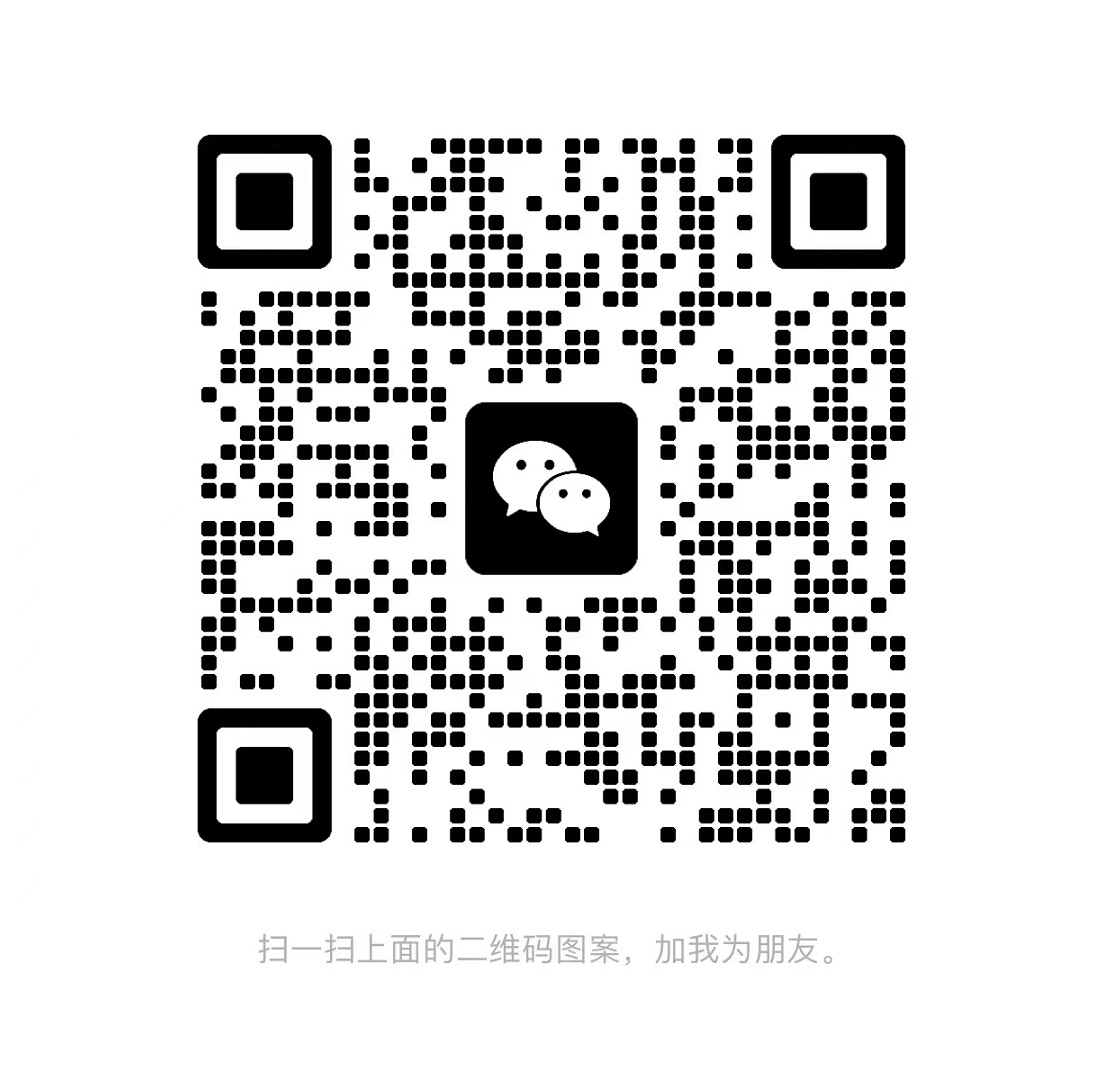