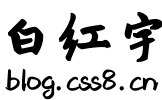
【MapReduce】基础案例 ---- Reduce Join 实现数据合并(表连接)
发布日期:2021-05-07 02:49:24
浏览次数:21
分类:精选文章
本文共 7862 字,大约阅读时间需要 26 分钟。
文章目录
一、Reduce Join
① Reduce Join工作原理
- Map端的主要工作:为来自不同表或文件的key/value对,打标签以区别不同来源的记录。然后用连接字段(公共字段)作为key,其余部分和新加的标志作为 value,最后进行输出。
- Reduce端的主要工作:在 Reduce端以连接字段作为key的分组已经完成,我们只需要在每一个分组当中将那些来源于不同文件的记录(在Map阶段已经打标志)分开,最后进行合并就ok了。
② Reduce Join 案例
☠ 需求
☠ 案例分析
通过将关联条件作为Map输出的key,将两表满足Join条件的数据并携带数据所来源的文件信息,发往同一个ReduceTask,在Reduce中进行数据的串联。
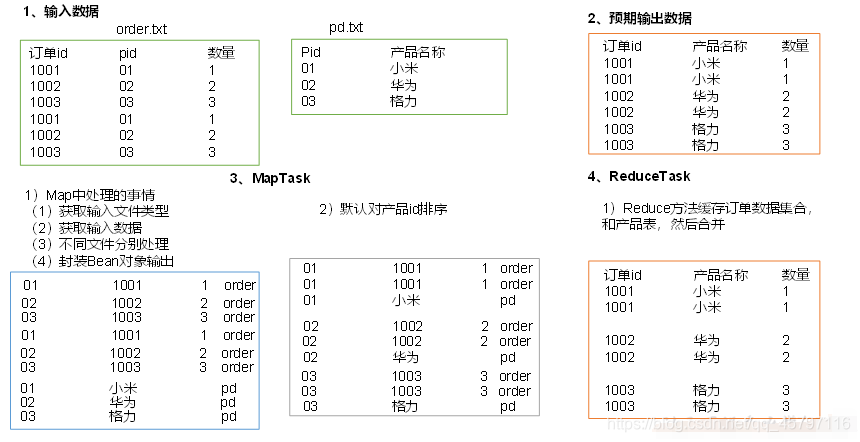
☠ 代码实现
封装Bean对象
package 第三章_MR框架原理.多种join应用;import org.apache.hadoop.io.Writable;import java.io.DataInput;import java.io.DataOutput;import java.io.IOException;public class TableBean implements Writable{ // id pid amount private String id; // 订单id private String pid;// 产品id private int amount;// 产品数量 // pid pname private String pname;// 产品名称 private String flag; // 定义一个标记,标记是订单表还是产品表 /** * 空参构造 */ public TableBean() { } /** * 有参构造 * @param id * @param pid * @param amount * @param pname * @param flag */ public TableBean(String id, String pid, int amount, String pname, String flag) { this.id = id; this.pid = pid; this.amount = amount; this.pname = pname; this.flag = flag; } /** * 序列化 * @param dataOutput * @throws IOException */ @Override public void write(DataOutput dataOutput) throws IOException { dataOutput.writeUTF(id); dataOutput.writeUTF(pid); dataOutput.writeInt(amount); dataOutput.writeUTF(pname); dataOutput.writeUTF(flag); } /** * 反序列化 * @param dataInput * @throws IOException */ @Override public void readFields(DataInput dataInput) throws IOException { id = dataInput.readUTF(); pid = dataInput.readUTF(); amount = dataInput.readInt(); pname = dataInput.readUTF(); flag = dataInput.readUTF(); } /** * 重写toString * @return */ @Override public String toString() { return id + '\t' + pname + '\t' + amount + '\''; } /** * set、get * @return */ public String getId() { return id; } public void setId(String id) { this.id = id; } public String getPid() { return pid; } public void setPid(String pid) { this.pid = pid; } public int getAmount() { return amount; } public void setAmount(int amount) { this.amount = amount; } public String getPname() { return pname; } public void setPname(String pname) { this.pname = pname; } public String getFlag() { return flag; } public void setFlag(String flag) { this.flag = flag; }}
Mapper阶段
与之前有所不同:
- 需要
重写setUp()方法
,通过切片信息获取文件名称
,判断读取的是哪个文件 map()方法中要对读取的文件判断处理
package 第三章_MR框架原理.多种join应用;import org.apache.hadoop.io.LongWritable;import org.apache.hadoop.io.Text;import org.apache.hadoop.mapreduce.Mapper;import org.apache.hadoop.mapreduce.lib.input.FileSplit;import java.io.IOException;public class TableMap extends Mapper{ String name; Text k = new Text(); TableBean v = new TableBean(); @Override protected void setup(Context context) throws IOException, InterruptedException { // 获取文件的名称 --- 通过切片信息获取 FileSplit inputSplit = (FileSplit) context.getInputSplit(); name = inputSplit.getPath().getName(); } @Override protected void map(LongWritable key, Text value, Context context) throws IOException, InterruptedException { // id pid amount // 1001 01 1 // pid pname // 01 小米 // 1.获取一行数据 String line = value.toString(); // 2.判断数据来源 if (name.startsWith("order")) { // 订单表数据 // 3.封装输出的key、value String[] fields = line.split("\t"); String id = fields[0]; String pid = fields[1]; int amount = Integer.parseInt(fields[2]); v.setId(id); v.setPid(pid); v.setAmount(amount); v.setPname(""); // 没有传入空字符串,但不能不写,因为序列化的时候有 v.setFlag("order"); // 设定标记 --- order表 k.set(pid); // 4.写出 context.write(k,v); } else { // 产品表数据 // 3.封装输出的key、value String[] fields = line.split("\t"); String pid = fields[0]; String pname = fields[1]; v.setId(""); // 没有传入空字符串,但不能不写,因为序列化的时候有 v.setPid(pid); // 没有传入默认值0 v.setAmount(0); v.setPname(pname); v.setFlag("opd"); // 设定标记 --- order表 k.set(pid); // 4.写出 context.write(k,v); } }}
Reducer阶段
package 第三章_MR框架原理.多种join应用;import org.apache.commons.beanutils.BeanUtils;import org.apache.hadoop.io.NullWritable;import org.apache.hadoop.io.Text;import org.apache.hadoop.mapreduce.Reducer;import java.io.IOException;import java.util.ArrayList;public class TableReduce extends Reducer{ @Override protected void reduce(Text key, Iterable values, Context context) throws IOException, InterruptedException { // 1.存储所有订单的集合 --- order ArrayList orderBeans = new ArrayList<>(); // 2.存储产品信息 --- pd TableBean pdBean = new TableBean(); // 3.遍历存储 for (TableBean value:values){ // 循环TableBean对象 if ("order".equals(value.getFlag())){ // 读取标记,判定为订单表 // 创建临时TableBean对象 TableBean temp = new TableBean(); try { // 将当前循环到的对象内容拷贝到临时TableBean对象 BeanUtils.copyProperties(temp,value); // 将其添加到集合中 orderBeans.add(temp); } catch (Exception e){ e.printStackTrace(); } }else{ // 读取标记,判定为产品表 try { // 将当前循环到的对象内容拷贝到 BeanUtils.copyProperties(pdBean,value); } catch (Exception e){ e.printStackTrace(); } } } // 4.将设置对应的产品名称,写出 for (TableBean tableBean:orderBeans){ tableBean.setPname(pdBean.getPname()); context.write(tableBean,NullWritable.get()); } }}
这里说明一下,为什么一个是集合,一个是对象:
- 相同的key可以同时进入到同一个reduce()中,在MapTask的时候对key进行了默认排序
- 如下图所示,一个reduce中只能获取01部分(或02或03)的数据集
- 而对应的每个部分中的order表数据不止一条,但是pd表数据只有一条,所以分别用集合、对象来存储。
Driver阶段
package 第三章_MR框架原理.多种join应用;import org.apache.hadoop.conf.Configuration;import org.apache.hadoop.fs.Path;import org.apache.hadoop.io.NullWritable;import org.apache.hadoop.io.Text;import org.apache.hadoop.mapreduce.Job;import org.apache.hadoop.mapreduce.lib.input.FileInputFormat;import org.apache.hadoop.mapreduce.lib.output.FileOutputFormat;public class TableDriver { public static void main(String[] args) { Job job = null; Configuration conf = new Configuration(); try{ // 1.获取job job = Job.getInstance(conf); // 2.配置 job.setMapperClass(TableMap.class); job.setReducerClass(TableReduce.class); job.setJarByClass(TableDriver.class); job.setMapOutputKeyClass(Text.class); job.setMapOutputValueClass(TableBean.class); job.setOutputKeyClass(TableBean.class); job.setOutputValueClass(NullWritable.class); // 设置输入输出路径 FileInputFormat.setInputPaths(job,new Path("G:\\Projects\\IdeaProject-C\\MapReduce\\src\\main\\java\\第三章_MR框架原理\\多种join应用\\data")); FileOutputFormat.setOutputPath(job,new Path("G:\\Projects\\IdeaProject-C\\MapReduce\\src\\main\\java\\第三章_MR框架原理\\多种join应用\\output")); // 提交job boolean result = job.waitForCompletion(true); System.exit(result? 0:1); } catch (Exception e){ e.printStackTrace(); } }}
☠ 总结
发表评论
最新留言
做的很好,不错不错
[***.243.131.199]2025年03月24日 18时34分32秒
关于作者
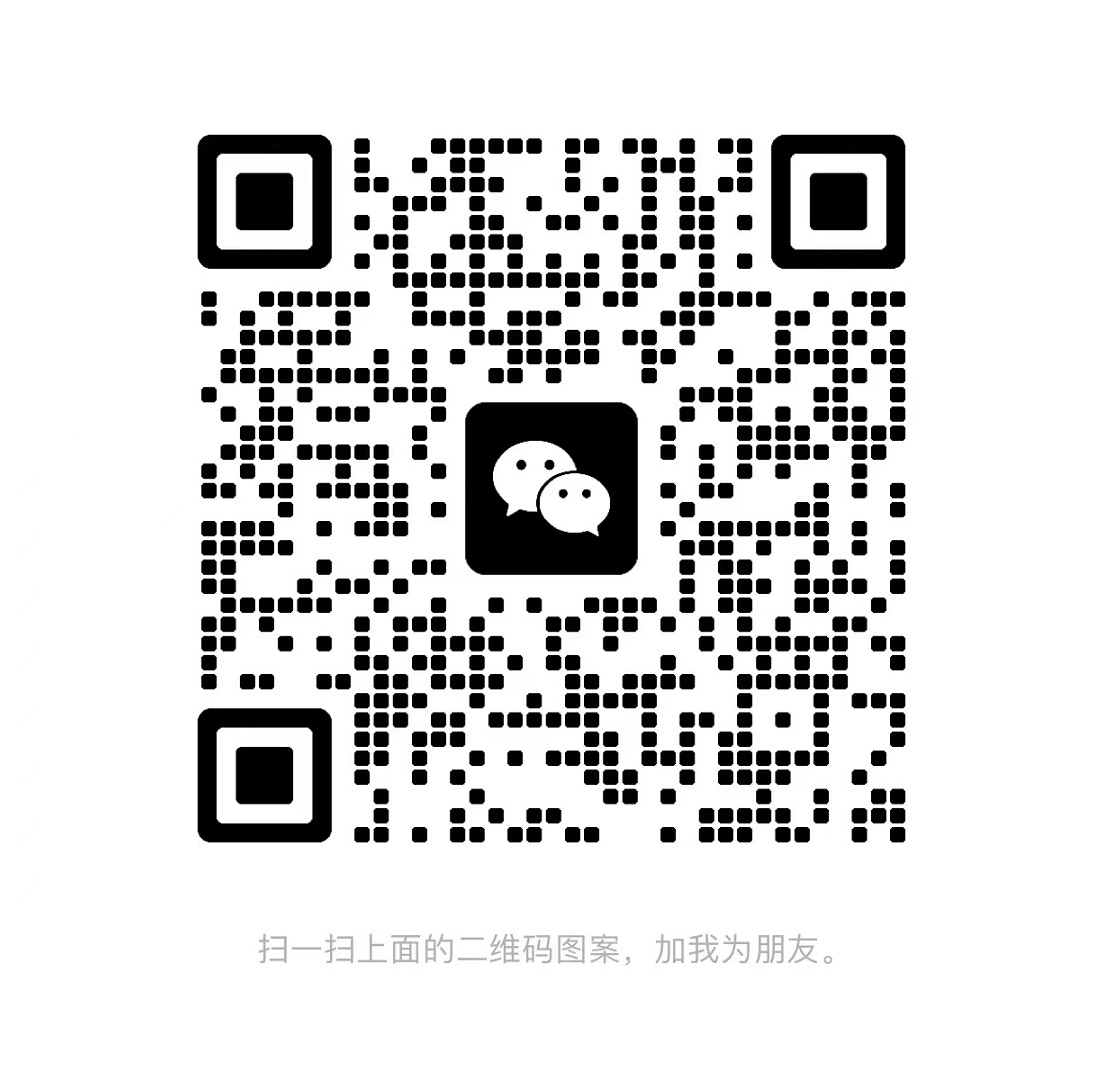
喝酒易醉,品茶养心,人生如梦,品茶悟道,何以解忧?唯有杜康!
-- 愿君每日到此一游!
推荐文章
程序员应该知道的97件事
2021-05-08
我编程,我快乐—程序员职业规划之道
2021-05-08
Web基础应用 NFS服务基础 触发挂载
2021-05-08
create-react-app路由的实现原理
2021-05-08
PSI值
2021-05-08
海思Hi3531DV100开发环境搭建
2021-05-08
JavaScript上传下载文件
2021-05-08
Linux驱动开发之PCIe Host驱动
2021-05-08
Vue.js Element Basic组件使用
2021-05-08
android 头像选择,裁剪全套解决方案,你值得拥有!
2021-05-08
MapReduce
2021-05-08
springboot swagger2
2021-05-08
shell(十)case的几个典型应用
2021-05-08
Linux环境变量配置错误导致命令不能使用(杂谈)
2021-05-08
openstack安装(六)镜像glance服务安装
2021-05-08
openstack安装(九)网络服务的安装--控制节点
2021-05-08
shell编程(六)语言编码规范之(变量)
2021-05-08
vim杂谈(三)之配色方案
2021-05-08
vim杂谈(五)之vim不加载~/.vimrc
2021-05-08
Linux杂谈之终端快捷键
2021-05-08