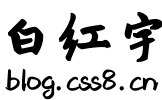
深度学习系列3:框架caffe
发布日期:2021-05-08 09:44:38
浏览次数:23
分类:精选文章
本文共 2680 字,大约阅读时间需要 8 分钟。
1. caffe介绍
caffe是贾扬清大神开发的一套系统,caffe2是重构后的版本。其基本结构为:
- 使用blob存储数据
- 使用operator连接blob数据,定义了计算规则
- 使用net组合operator形成批次计算的层
- 使用workspace管理网络、计算符和数据
2. 基本操作
2.1 数据
import numpy as npimport timefrom caffe2.python import core, workspacefrom caffe2.proto import caffe2_pb2X = np.random.randn(2, 3).astype(np.float32)print("Generated X from numpy:\n{}".format(X))workspace.FeedBlob("X", X)print(workspace.Blobs())print(workspace.FetchBlob('X'))
Blobs命令得到数据名列表
FetchBlob获得具体的数据2.2 操作符
op = core.CreateOperator( "Relu", # The type of operator that we want to run ["X"], # A list of input blobs by their names ["Y"], # A list of output blobs by their names 'active1')workspace.RunOperatorOnce(op)workspace.FetchBlobs('Y')op2 = core.CreateOperator( "GaussianFill", [], # GaussianFill does not need any parameters. ["W"], shape=[100, 100], # shape argument as a list of ints. mean=1.0, # mean as a single float std=1.0, # std as a single float)workspace.RunOperatorOnce(op2)temp = workspace.FetchBlob("Z")
使用workspace来控制运行。
2.3 网络
net = core.Net("linear_net")X = net.GaussianFill([], ["X"], mean=0.0, std=1.0, shape=[2, 3], run_once=0)W = net.GaussianFill([], ["W"], mean=0.0, std=1.0, shape=[5, 3], run_once=0)b = net.ConstantFill([], ["b"], shape=[5,], value=1.0, run_once=0)Y = net.FC([X,W,b],['Y'])print(net.Proto())workspace.RunNetOnce(net)for name in workspace.Blobs(): print("{}:\n{}".format(name, workspace.FetchBlob(name)))
在python中有两种方法来运行一个net:
方法1:使用workspace.RunNetOnce,初始化网络,运行网络,然后销毁网络。 方法2:先使用workspace.CreateNet初始化网络,然后使用workspace.RunNet来运行网络。 可以用下面的代码来画图:from caffe2.python import net_drawerfrom IPython import displaygraph = net_drawer.GetPydotGraph(net, rankdir="LR")display.Image(graph.create_png(), width=400)
2.4 运行推理
with open(INIT_NET) as f: init_net = f.read()with open(PREDICT_NET) as f: predict_net = f.read()p = workspace.Predictor(init_net, predict_net)results = p.run([img])
2.5 brew包
caffe2为了更方便调用和管理,可以通过导入brew这个包来调用帮助函数。像fc层的实现就可以使用:
from caffe2.python import brewbrew.fc(model, blob_in, blob_out, ...)
我们使用brew构造网络就十分简单,下面的代码就构造了一个LeNet模型:
from caffe2.python import brewdef AddLeNetModel(model, data): conv1 = brew.conv(model, data, 'conv1', 1, 20, 5) pool1 = brew.max_pool(model, conv1, 'pool1', kernel=2, stride=2) conv2 = brew.conv(model, pool1, 'conv2', 20, 50, 5) pool2 = brew.max_pool(model, conv2, 'pool2', kernel=2, stride=2) fc3 = brew.fc(model, pool2, 'fc3', 50 * 4 * 4, 500) fc3 = brew.relu(model, fc3, fc3) pred = brew.fc(model, fc3, 'pred', 500, 10) softmax = brew.softmax(model, pred, 'softmax')
caffe2 使用brew提供很多构造网络的帮助函数,大大简化了我们构建网络的过程。但实际上,这些只是封装的结果,网络构造的原理和之前说的使用operators构建的原理是一样的。
发表评论
最新留言
哈哈,博客排版真的漂亮呢~
[***.90.31.176]2025年04月08日 08时55分07秒
关于作者
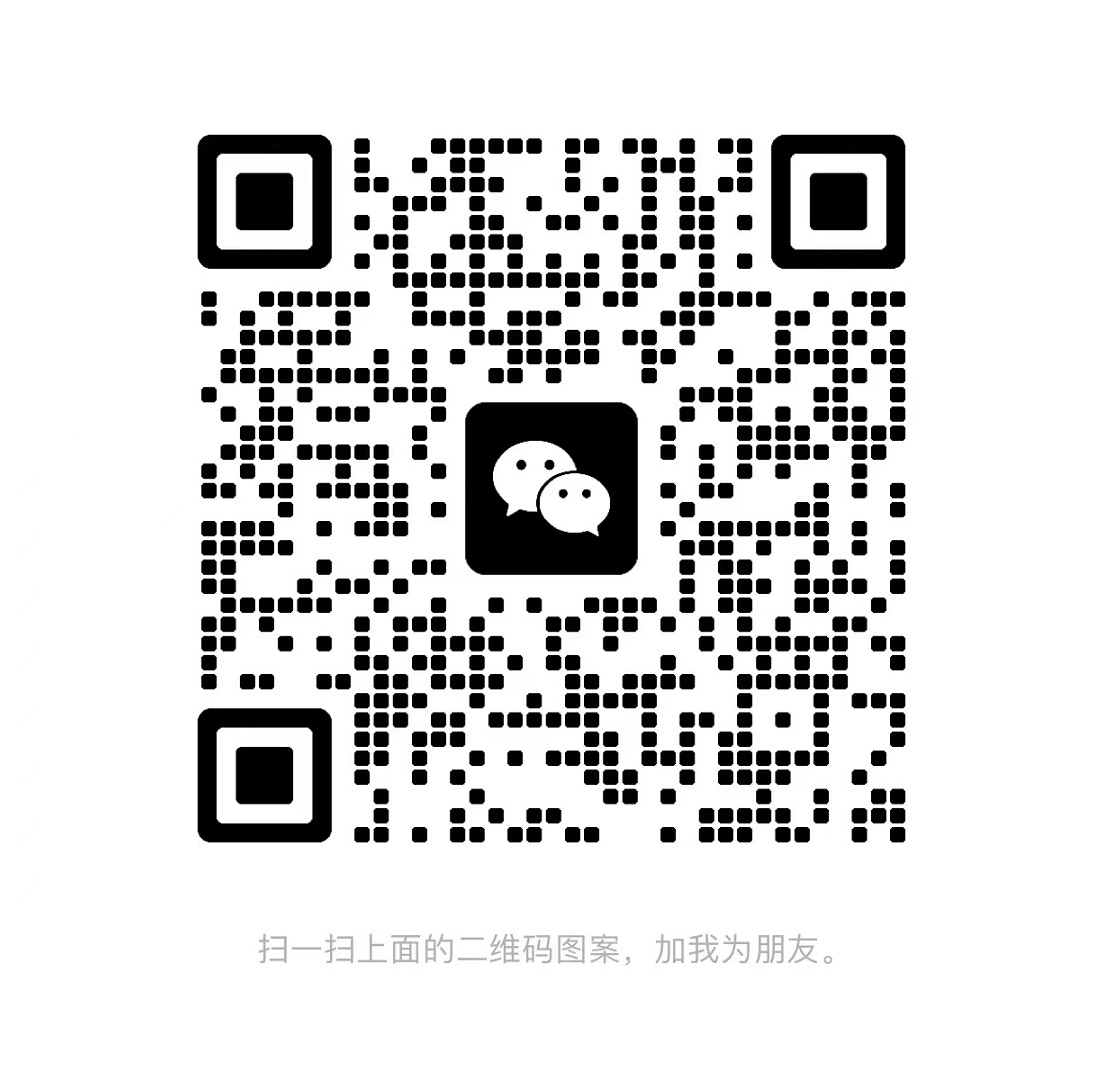
喝酒易醉,品茶养心,人生如梦,品茶悟道,何以解忧?唯有杜康!
-- 愿君每日到此一游!
推荐文章
abp(net core)+easyui+efcore实现仓储管理系统——多语言(十)
2021-05-09
WCF学习之旅—第三个示例之一(二十七)
2021-05-09
java ThreadPoolExecutor初探
2021-05-09
Markdown进阶
2021-05-09
快速指数算法
2021-05-09
python去除字符串中的特殊字符(爬虫存储数据时会遇到不能作为文件名的字符串)
2021-05-09
PHP将网址快捷方式保存到桌面
2021-05-09
SpringCloud微服务(03):Hystrix组件,实现服务熔断
2021-05-09
Spring 框架基础(01):核心组件总结,基础环境搭建
2021-05-09
JavaEE基础(02):Servlet核心API用法详解
2021-05-09
SpringBoot2 整合Nacos组件,环境搭建和入门案例详解
2021-05-09
结构与算法(03):单向链表和双向链表
2021-05-09
Hadoop框架:MapReduce基本原理和入门案例
2021-05-09
ThreadPoolExecutor线程池任务执行失败的时候会怎样
2021-05-09
Sentry快速开始并集成钉钉群机器人
2021-05-09
Docker 服务
2021-05-09
第一眼就心动的人还怎么做朋友
2021-05-09