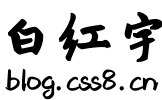
本文共 4125 字,大约阅读时间需要 13 分钟。
《用于自然状态下人脸去遮挡的遮挡发现GAN》
声明
本文仅为学习用途,原文作者为Jiayuan Dong1, Liyan Zhang1, Hanwang Zhang2, Weichen Liu2。
摘要
被遮挡的人脸是现实生活中常见的场景,却对大多数人脸识别系统造成显著负面影响。现有的方法尝试通过单一生成对抗性网络(GAN)消除遮挡,但其无法识别多种遮挡类型。为此,我们提出两阶段遮挡识别GAN(OA-GAN),其中第一阶段用于分离遮挡,第二阶段基于遮挡生成无遮挡人脸。实验结果表明,OA-GAN显著优于现有方法,且可提高遮挡下面部表情识别(FER)的准确性。
引言
遮挡对人脸识别系统的影响尤为严重,尤其在实际应用中,遮挡物体的形状、位置及类型多样,现有方法难以应对复杂的真实世界遮挡场景。传统方法通常通过单一GAN直接生成无遮挡人脸,但其效果有限,且难以推广至多样化遮挡情境。
我们提出两阶段遮挡识别GAN(OA-GAN),通过分离遮挡物体生成更精确的无遮挡人脸。这种两阶段模型不仅能够处理不同类型的遮挡,还能更好地解释生成过程。
OA-GAN:两阶段遮挡感知的GAN
OA-GAN由两个生成器(G1和G2)和两个判别器(D1和D2)组成。其工作流程如下:
关键创新点:
- OA-GAN采用U-Net架构,确保生成器鲁棒性强。
- 马尔可夫判别器用于局部惩罚,避免全局模糊。
- 针对遮挡合成,引入像素级L1损失,提升图像清晰度。
实验
数据集
实验细节
- CelebA数据集结合细节:生成四组图像集合,区分是否看到人脸或遮挡。
- 网络架构:基于pix2pix构建单级网络和OA-GAN,λ1和λ2均设为100。
视觉分析
图4展示了CK+和CelebA数据集上合成的遮挡和无遮挡脸结果,图5显示真实世界图像合成的失败案例。实验表明,OA-GAN生成的遮挡图像与无遮挡图像高度相关,且遮挡合成是关键环节。
定量分析
- 去遮挡率:inception v3模型在CelebA数据集上训练,准确度达到0.9996。
- PSNR和SSIM:OA-GAN生成图像在清晰度和相似性上优于传统方法。
面部表情识别
通过FER任务验证,OA-GAN显著提高遮挡下模型的性能,表明去遮挡过程保留了关键表达信息。
结论
OA-GAN通过两阶段设计显式消除面部遮挡,能够处理复杂的真实世界遮挡情境。实验表明,其性能优于现有方法,且支持后续研究如面部表情识别。
参考文献
[1] John Wright, Allen Y Yang, Arvind Ganesh, S Shankar Sastry, and Yi Ma, “Robust face cognition via sparse representation,” IEEE transactions on pattern analysis and machine intelligence, vol. 31, no. 2, pp. 210–227, 2008.
[2] Joe Mathai, Iacopo Masi, and Wael AbdAlmageed, “Does generative face completion help ace recognition?,” arXiv preprint arXiv:1906.02858, 2019. [3] Fang Zhao, Jiashi Feng, Jian Zhao, Wenhan Yang, and Shuicheng Yan, “Robust stm-autoencoders for face deocclusion in the wild,” IEEE Transactions on Image Processing, vol. 7, no. 2, pp. 778–790, 2017. [4] Xiaohua Xie, Wei-Shi Zheng, Jianhuang Lai, Pong C Yuen, and Ching Y Suen, Normalization of face illumination based on large-and small-scale features,” IEEE Transactions on Image Processing, vol. 20, no. 7, pp.1807–1821, 2010. [5] Yichen Qian, Weihong Deng, and Jiani Hu, “Unsupervised face normalization with extreme pose and expression in the wild,” in Proceedings of the IEEE Conference on Computer Vision and Pattern Recognition, 2019, pp.9851–9858. [6] Ligang Zhang, Brijesh Verma, Dian Tjondronegoro, and Vinod Chandran, “Facial expression analysis under partial occlusion: A survey,” ACM Computing Surveys(CSUR), vol. 51, no. 2, pp. 25, 2018. [7] Mehdi Mirza and Simon Osindero, “Conditional generative adversarial nets,” arXiv preprint arXiv:1411.1784, 2014. [8] Jiancheng Cai, Han Hu, Shiguang Shan, and Xilin Chen, “Fcsr-gan: End-to-end learning for joint face completion and super-resolution,” in 2019 14th IEEE International Conference on Automatic Face & Gesture Recognition (FG 2019). IEEE, 2019, pp. 1–8. [9] Yijun Li, Sifei Liu, Jimei Yang, and Ming-Hsuan Yang, “Generative face completion,” in Proceedings of the IEEE Conference on Computer Vision and Pattern Recognition, 2017, pp. 3911–3919. [10] Chuanxia Zheng, Tat-Jen Cham, and Jianfei Cai, “Pluralistic image completion,” in Proceedings of the IEEE Conference on Computer Vision and Pattern Recognition, 2019, pp. 1438–1447. [11] Olaf Ronneberger, Philipp Fischer, and Thomas Brox, “U-net: Convolutional networks for biomedical image segmentation,” in International Conference on Medical image computing and computer-assisted intervention. Springer, 2015, pp. 234–241. [12] Phillip Isola, Jun-Yan Zhu, Tinghui Zhou, and Alexei AEfros, “Image-to-image translation with conditional adversarial networks,” in Proceedings of the IEEE conference on computer vision and pattern recognition, 2017, pp. 1125–1134. [13] Patrick Lucey, Jeffrey F Cohn, Takeo Kanade, Jason Saragih, Zara Ambadar, and Iain Matthews, “The extended cohn-kanade dataset (ck+): A complete dataset for action unit and emotion-specifified expression,” in 2010 IEEE Computer Society Conference on Computer Vision and Pattern Recognition-Workshops. IEEE, 2010, pp. 94–101. [14] Ziwei Liu, Ping Luo, Xiaogang Wang, and Xiaoou Tang, “Deep learning face attributes in the wild,” in Proceedings of International Conference on Computer Vision (ICCV), December 2015.发表评论
最新留言
关于作者
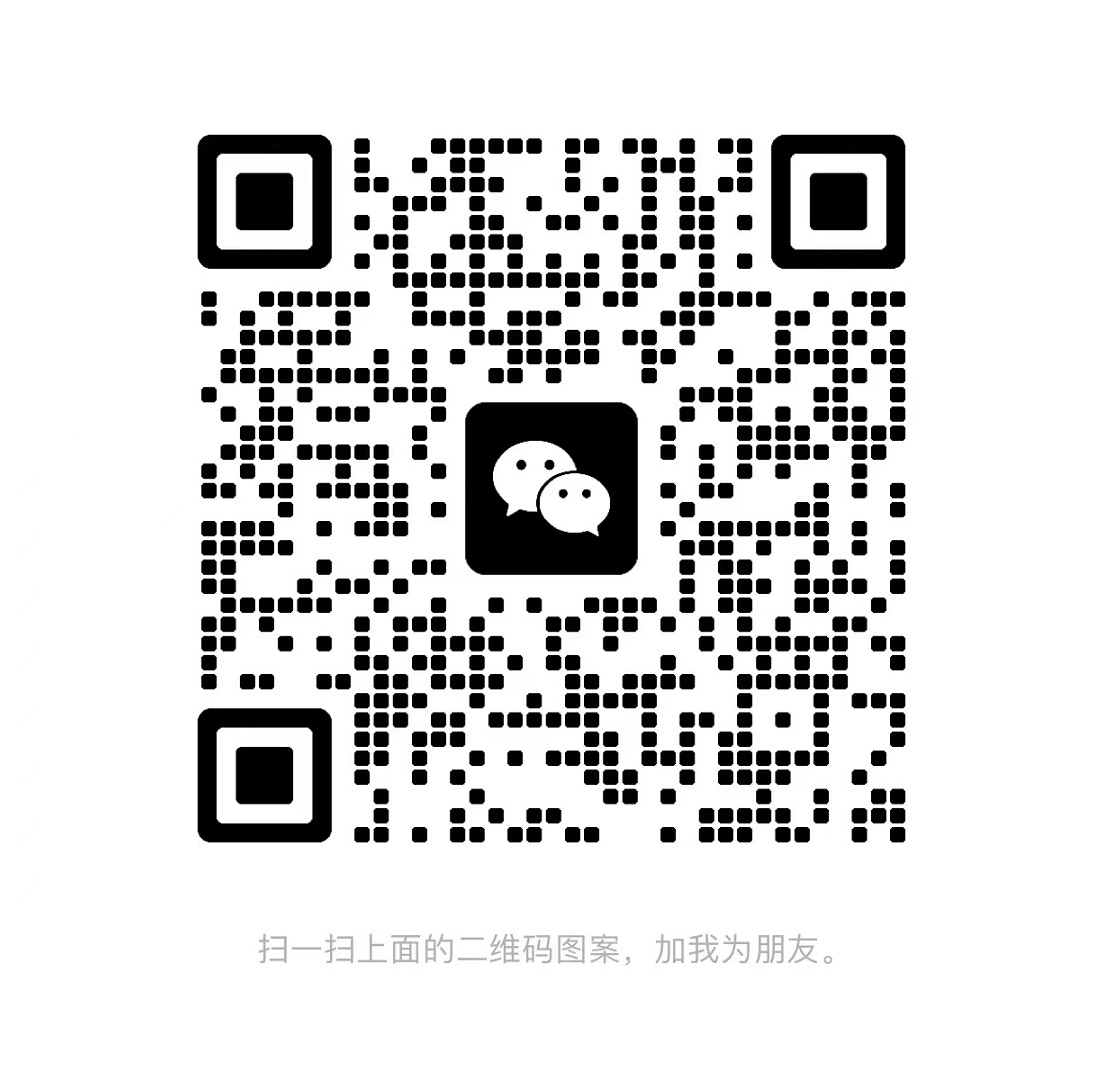