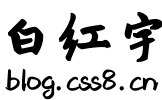
本文共 3285 字,大约阅读时间需要 10 分钟。
-
class TfidfVectorizer
class sklearn.feature_extraction.text.TfidfVectorizer(input=’content’, encoding=’utf-8’, decode_error=’strict’, strip_accents=None, lowercase=True, preprocessor=None, tokenizer=None, analyzer=’word’, stop_words=None, token_pattern=’(?u)\b\w\w+\b’, ngram_range=(1, 1), max_df=1.0, min_df=1, max_features=None, vocabulary=None, binary=False, dtype=<class ‘numpy.float64’>, norm=’l2’, use_idf=True, smooth_idf=True, sublinear_tf=False)
将原始文档转化成TF-IDF特征表示的矩阵。
等价于之后再进行
更多内容参见:
-
Parameters
Parameters | 数据类型 | 意义 |
---|---|---|
input | string {‘filename’, ‘file’, ‘content’} | 待处理对象 |
encoding | string, ‘utf-8’ by default. | 解码方式 |
decode_error | {‘strict’, ‘ignore’, ‘replace’} | 如果处理字节文件,而文件中包含给定encoding 解码失败的字符,指示程序如何处理,默认strict ,返回一个UnicodeDecodeError 。 |
strip_accents | {‘ascii’, ‘unicode’, None} | 预处理(preprocessing)阶段取出语料中的重音符号。 ‘ascii’:速度快,只严格匹配ASCII; ’unicode‘:稍慢,匹配所有字符 None:default不做任何处理 |
lowercase | boolean | 标记之前,把所有字符转成小写 |
preprocessor | callable or None (default) | 覆盖预处理阶段,但是保留标记(tokenizing)和n-grams生成步骤 |
tokenizer | callable or None (default) | 覆盖tokenization,保留预处理和n-grams生成步骤。只有在analyzer == 'word' 时使用 |
stop_words | string {‘english’}, list, or None (default) | ‘english’:使用内置的英语停止词 list:自定义停止词 None:没有停止词 |
token_pattern | string | 构成token的正则表达式,只在analyzer == 'word' 时使用,默认规则选择2个或以上字母或数字字符,忽略标点,且标点作为token分隔器 |
ngram_range | tuple (min_n, max_n) | n-grams提取中n值的上下界,界内所有n值(min_n <= n <= max_n)都会被用到 |
analyzer | string, {‘word’, ‘char’, ‘char_wb’} or callable | Whether the feature should be made of word or character n-grams. Option ‘char_wb’ creates character n-grams only from text inside word boundaries; n-grams at the edges of words are padded with space. If a callable is passed it is used to extract the sequence of features out of the raw, unprocessed input. |
max_df | float in range [0.0, 1.0] or int, default=1.0 | 创建词汇表时,忽略超过给定阈值的项目。 float:出现次数与语料库总数比例 int:绝对计数 如果给定vocabulary参数,则此参数忽略 |
min_df | specific | 同上,下界 |
max_features | int or None, default=None | vocabulary如果是Not None:忽略此参数 ;如果不是None:整个语料库(corpus)按频率排列,取max_features个特征 |
vocabulary | Mapping or iterable, optional | r如果没给定参数:vocabulary由输入文档决定 Mapping:在特征矩阵中,键是terms,值是indices iterable: |
binary | boolean,False(Defalt) | True:所有非零计数设置为1,用于二元事件的离散概率模型 |
dtype | type,optional | fit_transform() or transform()返回的矩阵类型 |
norm | ‘l1’, ‘l2’ or None, optional (default=’l2’) | 正则化 |
use_idf | boolean (default=True) | 启用inverse-document-frequency重赋权重 |
smooth_idf | boolean (default=True) | 平滑idf |
sublinear_tf | boolean (default=False) | 用1 + log(tf) 替换tf ,实现亚线性 |
-
Attributes
Parameter | 数据类型 | 意义 |
---|---|---|
vocabulary_ | dict | A mapping of terms to feature indices. |
idf_ | array, shape (n_features) | idf向量 |
stop_words_ | set | 停止词 |
-
方法Methods
-
(self)
返回一个callable,用于预处理和标注
-
(self)
返回一个函数,用在标注之前对text预处理
-
(self)
返回一个函数,将字符串切分成tokens序列
-
(self, doc)
将输入解码成unicode符。
doc,需要decode的字符串
-
(self, raw_documents[, y])
从原始文件中学出一个字典结构的全部tokens的词汇表
-
(self, raw_documents[, y])
学出字典结构词汇表,返回一个term-document矩阵。
等价于transform之后fit,不过更高效
-
(self)
一个从特征证书指标映射到特征名字的数组
-
(self[, deep])
得到评估量的参数
-
(self)
创建或获取有效的停止词列表
-
(self, X)
返回X中每个有非零词目的文件。(X_inv : list of arrays, len = n_samples)
X : {array, sparse matrix}, shape = [n_samples, n_features]
-
(self, **params)
设置这个评估器的参数
-
(self, raw_documents)
将文件转换成document-term矩阵。
用经由fit拟合的词汇表或给定的构造函数,从原始text文件中提取token数量。
raw_documents : iterable str, unicode or file objects都可以
X : sparse matrix, [n_samples, n_features] Document-term matrix。
-
发表评论
最新留言
关于作者
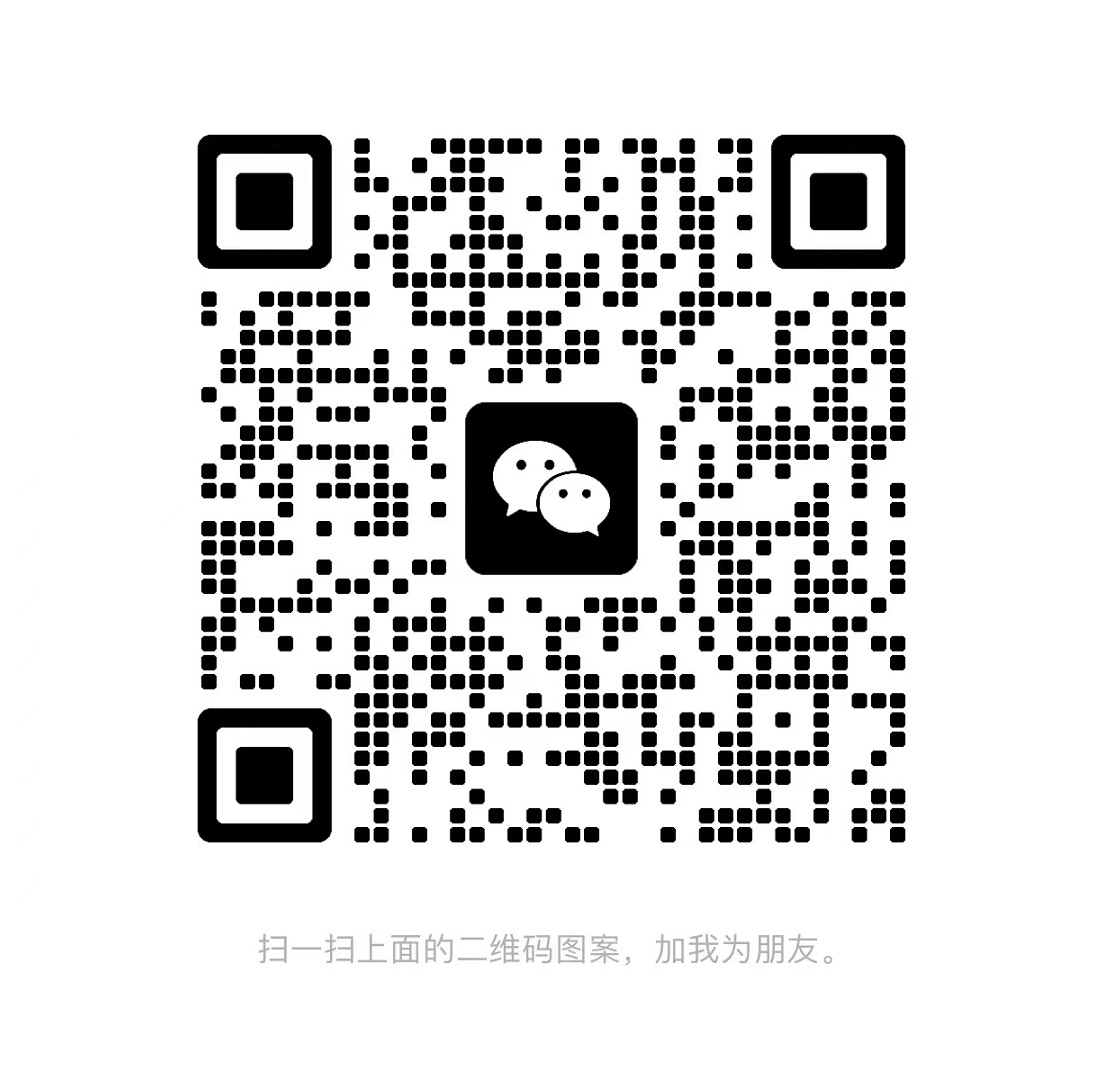