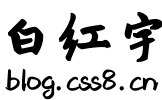
numpy+cnn
发布日期:2021-05-06 21:48:23
浏览次数:22
分类:技术文章
本文共 10446 字,大约阅读时间需要 34 分钟。
import numpyimport sysdef conv_(img, conv_filter): filter_size = conv_filter.shape[1] result = numpy.zeros((img.shape)) #Looping through the image to apply the convolution operation. for r in numpy.uint16(numpy.arange(filter_size/2.0, img.shape[0]-filter_size/2.0+1)): for c in numpy.uint16(numpy.arange(filter_size/2.0, img.shape[1]-filter_size/2.0+1)): """ Getting the current region to get multiplied with the filter. How to loop through the image and get the region based on the image and filer sizes is the most tricky part of convolution. """ curr_region = img[r-numpy.uint16(numpy.floor(filter_size/2.0)):r+numpy.uint16(numpy.ceil(filter_size/2.0)), c-numpy.uint16(numpy.floor(filter_size/2.0)):c+numpy.uint16(numpy.ceil(filter_size/2.0))] #Element-wise multipliplication between the current region and the filter. curr_result = curr_region * conv_filter conv_sum = numpy.sum(curr_result) #Summing the result of multiplication. result[r, c] = conv_sum #Saving the summation in the convolution layer feature map. #Clipping the outliers of the result matrix. final_result = result[numpy.uint16(filter_size/2.0):result.shape[0]-numpy.uint16(filter_size/2.0), numpy.uint16(filter_size/2.0):result.shape[1]-numpy.uint16(filter_size/2.0)] return final_resultdef conv(img, conv_filter): if len(img.shape) > 2 or len(conv_filter.shape) > 3: # Check if number of image channels matches the filter depth. if img.shape[-1] != conv_filter.shape[-1]: print("Error: Number of channels in both image and filter must match.") sys.exit() if conv_filter.shape[1] != conv_filter.shape[2]: # Check if filter dimensions are equal. print('Error: Filter must be a square matrix. I.e. number of rows and columns must match.') sys.exit() if conv_filter.shape[1]%2==0: # Check if filter diemnsions are odd. print('Error: Filter must have an odd size. I.e. number of rows and columns must be odd.') sys.exit() # An empty feature map to hold the output of convolving the filter(s) with the image. feature_maps = numpy.zeros((img.shape[0]-conv_filter.shape[1]+1, img.shape[1]-conv_filter.shape[1]+1, conv_filter.shape[0])) # Convolving the image by the filter(s). for filter_num in range(conv_filter.shape[0]): print("Filter ", filter_num + 1) curr_filter = conv_filter[filter_num, :] # getting a filter from the bank. """ Checking if there are mutliple channels for the single filter. If so, then each channel will convolve the image. The result of all convolutions are summed to return a single feature map. """ if len(curr_filter.shape) > 2: conv_map = conv_(img[:, :, 0], curr_filter[:, :, 0]) # Array holding the sum of all feature maps. for ch_num in range(1, curr_filter.shape[-1]): # Convolving each channel with the image and summing the results. conv_map = conv_map + conv_(img[:, :, ch_num], curr_filter[:, :, ch_num]) else: # There is just a single channel in the filter. conv_map = conv_(img, curr_filter) feature_maps[:, :, filter_num] = conv_map # Holding feature map with the current filter. return feature_maps # Returning all feature maps. def pooling(feature_map, size=2, stride=2): #Preparing the output of the pooling operation. pool_out = numpy.zeros((numpy.uint16((feature_map.shape[0]-size+1)/stride+1), numpy.uint16((feature_map.shape[1]-size+1)/stride+1), feature_map.shape[-1])) for map_num in range(feature_map.shape[-1]): r2 = 0 for r in numpy.arange(0,feature_map.shape[0]-size+1, stride): c2 = 0 for c in numpy.arange(0, feature_map.shape[1]-size+1, stride): pool_out[r2, c2, map_num] = numpy.max([feature_map[r:r+size, c:c+size, map_num]]) c2 = c2 + 1 r2 = r2 +1 return pool_outdef relu(feature_map): #Preparing the output of the ReLU activation function. relu_out = numpy.zeros(feature_map.shape) for map_num in range(feature_map.shape[-1]): for r in numpy.arange(0,feature_map.shape[0]): for c in numpy.arange(0, feature_map.shape[1]): relu_out[r, c, map_num] = numpy.max([feature_map[r, c, map_num], 0]) return relu_out
import skimage.dataimport numpyimport matplotlibimport NumPyCNN as numpycnn# Reading the image#img = skimage.io.imread("test.jpg")#img = skimage.data.checkerboard()img = skimage.data.chelsea()#img = skimage.data.camera()# Converting the image into gray.img = skimage.color.rgb2gray(img)# First conv layer#l1_filter = numpy.random.rand(2,7,7)*20 # Preparing the filters randomly.l1_filter = numpy.zeros((2,3,3))l1_filter[0, :, :] = numpy.array([[[-1, 0, 1], [-1, 0, 1], [-1, 0, 1]]])l1_filter[1, :, :] = numpy.array([[[1, 1, 1], [0, 0, 0], [-1, -1, -1]]])print("\n**Working with conv layer 1**")l1_feature_map = numpycnn.conv(img, l1_filter)print("\n**ReLU**")l1_feature_map_relu = numpycnn.relu(l1_feature_map)print("\n**Pooling**")l1_feature_map_relu_pool = numpycnn.pooling(l1_feature_map_relu, 2, 2)print("**End of conv layer 1**\n")# Second conv layerl2_filter = numpy.random.rand(3, 5, 5, l1_feature_map_relu_pool.shape[-1])print("\n**Working with conv layer 2**")l2_feature_map = numpycnn.conv(l1_feature_map_relu_pool, l2_filter)print("\n**ReLU**")l2_feature_map_relu = numpycnn.relu(l2_feature_map)print("\n**Pooling**")l2_feature_map_relu_pool = numpycnn.pooling(l2_feature_map_relu, 2, 2)print("**End of conv layer 2**\n")# Third conv layerl3_filter = numpy.random.rand(1, 7, 7, l2_feature_map_relu_pool.shape[-1])print("\n**Working with conv layer 3**")l3_feature_map = numpycnn.conv(l2_feature_map_relu_pool, l3_filter)print("\n**ReLU**")l3_feature_map_relu = numpycnn.relu(l3_feature_map)print("\n**Pooling**")l3_feature_map_relu_pool = numpycnn.pooling(l3_feature_map_relu, 2, 2)print("**End of conv layer 3**\n")# Graphing resultsfig0, ax0 = matplotlib.pyplot.subplots(nrows=1, ncols=1)ax0.imshow(img).set_cmap("gray")ax0.set_title("Input Image")ax0.get_xaxis().set_ticks([])ax0.get_yaxis().set_ticks([])matplotlib.pyplot.savefig("in_img.png", bbox_inches="tight")matplotlib.pyplot.close(fig0)# Layer 1fig1, ax1 = matplotlib.pyplot.subplots(nrows=3, ncols=2)ax1[0, 0].imshow(l1_feature_map[:, :, 0]).set_cmap("gray")ax1[0, 0].get_xaxis().set_ticks([])ax1[0, 0].get_yaxis().set_ticks([])ax1[0, 0].set_title("L1-Map1")ax1[0, 1].imshow(l1_feature_map[:, :, 1]).set_cmap("gray")ax1[0, 1].get_xaxis().set_ticks([])ax1[0, 1].get_yaxis().set_ticks([])ax1[0, 1].set_title("L1-Map2")ax1[1, 0].imshow(l1_feature_map_relu[:, :, 0]).set_cmap("gray")ax1[1, 0].get_xaxis().set_ticks([])ax1[1, 0].get_yaxis().set_ticks([])ax1[1, 0].set_title("L1-Map1ReLU")ax1[1, 1].imshow(l1_feature_map_relu[:, :, 1]).set_cmap("gray")ax1[1, 1].get_xaxis().set_ticks([])ax1[1, 1].get_yaxis().set_ticks([])ax1[1, 1].set_title("L1-Map2ReLU")ax1[2, 0].imshow(l1_feature_map_relu_pool[:, :, 0]).set_cmap("gray")ax1[2, 0].get_xaxis().set_ticks([])ax1[2, 0].get_yaxis().set_ticks([])ax1[2, 0].set_title("L1-Map1ReLUPool")ax1[2, 1].imshow(l1_feature_map_relu_pool[:, :, 1]).set_cmap("gray")ax1[2, 0].get_xaxis().set_ticks([])ax1[2, 0].get_yaxis().set_ticks([])ax1[2, 1].set_title("L1-Map2ReLUPool")matplotlib.pyplot.savefig("L1.png", bbox_inches="tight")matplotlib.pyplot.close(fig1)# Layer 2fig2, ax2 = matplotlib.pyplot.subplots(nrows=3, ncols=3)ax2[0, 0].imshow(l2_feature_map[:, :, 0]).set_cmap("gray")ax2[0, 0].get_xaxis().set_ticks([])ax2[0, 0].get_yaxis().set_ticks([])ax2[0, 0].set_title("L2-Map1")ax2[0, 1].imshow(l2_feature_map[:, :, 1]).set_cmap("gray")ax2[0, 1].get_xaxis().set_ticks([])ax2[0, 1].get_yaxis().set_ticks([])ax2[0, 1].set_title("L2-Map2")ax2[0, 2].imshow(l2_feature_map[:, :, 2]).set_cmap("gray")ax2[0, 2].get_xaxis().set_ticks([])ax2[0, 2].get_yaxis().set_ticks([])ax2[0, 2].set_title("L2-Map3")ax2[1, 0].imshow(l2_feature_map_relu[:, :, 0]).set_cmap("gray")ax2[1, 0].get_xaxis().set_ticks([])ax2[1, 0].get_yaxis().set_ticks([])ax2[1, 0].set_title("L2-Map1ReLU")ax2[1, 1].imshow(l2_feature_map_relu[:, :, 1]).set_cmap("gray")ax2[1, 1].get_xaxis().set_ticks([])ax2[1, 1].get_yaxis().set_ticks([])ax2[1, 1].set_title("L2-Map2ReLU")ax2[1, 2].imshow(l2_feature_map_relu[:, :, 2]).set_cmap("gray")ax2[1, 2].get_xaxis().set_ticks([])ax2[1, 2].get_yaxis().set_ticks([])ax2[1, 2].set_title("L2-Map3ReLU")ax2[2, 0].imshow(l2_feature_map_relu_pool[:, :, 0]).set_cmap("gray")ax2[2, 0].get_xaxis().set_ticks([])ax2[2, 0].get_yaxis().set_ticks([])ax2[2, 0].set_title("L2-Map1ReLUPool")ax2[2, 1].imshow(l2_feature_map_relu_pool[:, :, 1]).set_cmap("gray")ax2[2, 1].get_xaxis().set_ticks([])ax2[2, 1].get_yaxis().set_ticks([])ax2[2, 1].set_title("L2-Map2ReLUPool")ax2[2, 2].imshow(l2_feature_map_relu_pool[:, :, 2]).set_cmap("gray")ax2[2, 2].get_xaxis().set_ticks([])ax2[2, 2].get_yaxis().set_ticks([])ax2[2, 2].set_title("L2-Map3ReLUPool")matplotlib.pyplot.savefig("L2.png", bbox_inches="tight")matplotlib.pyplot.close(fig2)# Layer 3fig3, ax3 = matplotlib.pyplot.subplots(nrows=1, ncols=3)ax3[0].imshow(l3_feature_map[:, :, 0]).set_cmap("gray")ax3[0].get_xaxis().set_ticks([])ax3[0].get_yaxis().set_ticks([])ax3[0].set_title("L3-Map1")ax3[1].imshow(l3_feature_map_relu[:, :, 0]).set_cmap("gray")ax3[1].get_xaxis().set_ticks([])ax3[1].get_yaxis().set_ticks([])ax3[1].set_title("L3-Map1ReLU")ax3[2].imshow(l3_feature_map_relu_pool[:, :, 0]).set_cmap("gray")ax3[2].get_xaxis().set_ticks([])ax3[2].get_yaxis().set_ticks([])ax3[2].set_title("L3-Map1ReLUPool")matplotlib.pyplot.savefig("L3.png", bbox_inches="tight")matplotlib.pyplot.close(fig3)
图片保存结果如下:
It's amazing!
卷积层-激活层-池化层
提问:像素全为正,relu激活层还有什么用呢?
答:卷积滤波器的参数可以为负,卷积层的输出可以是负值,所以relu激活层仍然有意义;
提问:池化层后不是就只有一个值了吗?
答:池化层和卷积层一样也有一个类似滤波器进行池化,进行了一个下采样,而不是对整个featuremap选择最大的一个值;比如说2*2 步长为2,每四个元素中选择最大的一个;
提问:为什么没有训练也会不错的效果呢?
答:从逆向思维的角度来看,说明卷积层参数初始值设置得不错;如图所示:本文中的滤波器值如下
发表评论
最新留言
路过按个爪印,很不错,赞一个!
[***.219.124.196]2025年03月14日 04时02分42秒
关于作者
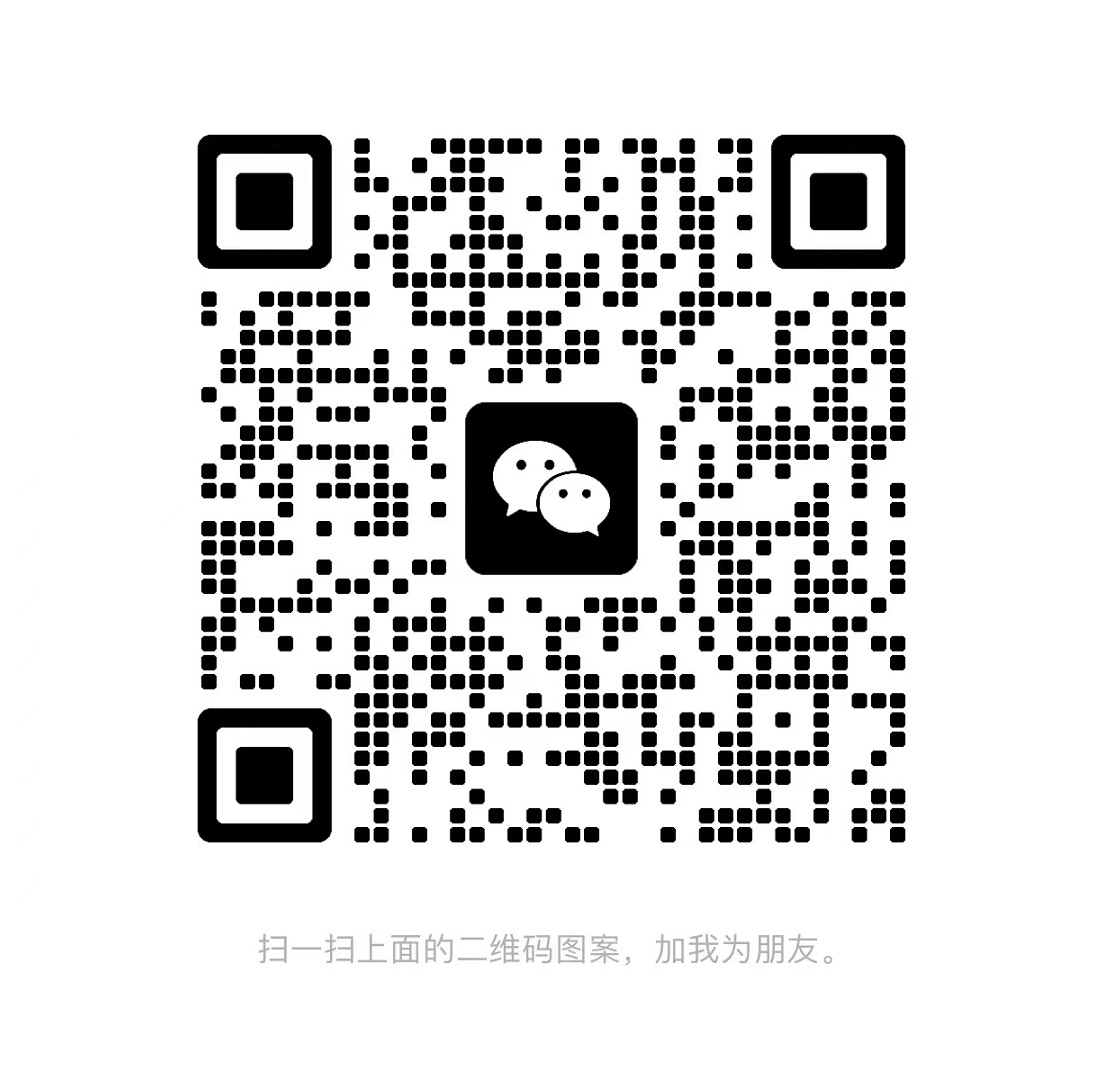
喝酒易醉,品茶养心,人生如梦,品茶悟道,何以解忧?唯有杜康!
-- 愿君每日到此一游!
推荐文章
详解: 最小生成树
2019-03-03
[编程题]:n头牛中选择满足所有m种特性的牛(百度2021)
2019-03-03
Redis中的删除策略和逐出算法
2019-03-03
[数据结构]:红黑树(二)
2019-03-03
PyCharm安装与配置
2019-03-03
关于Java的List的笔记
2019-03-03
Docker - 部署 Redis 6.0.8
2019-03-03
Android - Broadcasts overview(不完整)
2019-03-03
OrCAD Capture CIS 16.6 - 为元器件添加属性
2019-03-03
排序算法总结——c++实现
2019-03-03
c++ 逗号运算符
2019-03-03
vector的几种初始化及赋值方式
2019-03-03
STL基础梳理 2019.1.19(仿函数,谓词,内建函数对象,适配器,算法)
2019-03-03
IDEA效率快捷键收集
2019-03-03
并发情况下三种线程/并发安全
2019-03-03
希尔排序
2019-03-03