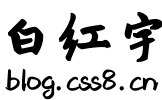
本文共 17468 字,大约阅读时间需要 58 分钟。
1. _load_gt_file函数:
从train3.txt或者val3.txt里面读取原图片和标签图片的地址,再用scipy.misc.imread(image_dir,mode='RGB')将图片读取出来并且以矩阵的形式存储。Take the data_file and hypes and create a generator. The generator outputs the image and the gt_image.
2. _make_data_gen函数:
如果是val那么直接返回原图,标签图片。注意这个标签图片不是原来的RGB图像而是只有两通道的二值图像(实际上就是bool型的),第一层是background(如果是背景则为True,不是则False;故白的是背景,黑的是其他),第二层是road(同理,是路则为True,不是则为False;故白的是路,黑的是其他)
如果是train,那么还需要对两种图像进行jitter也就是图像的增强之类的。然后返回处理之后的图像。
3.jitter_input 函数:
统领所有的图像增强方法
图像增强:
1)random_crop 随机裁剪索。所要裁剪的图片是原图的一部分。随机裁剪指的是裁剪的位置是随机的,这个随机也是在满足裁剪的大小固定的前提下的也就是说随机的位置是在一定的范围内,当原图进行裁剪了,标签图片也要进行相同位置的裁剪出相同的大小。
2)random_crop_soft 是另外一种裁剪方式,裁剪位置是随机的,裁剪大小也是随机的。随机的位置为offset。一半的概率是从裁剪位置offset开始到最后为裁剪图片后的大小,另外一半的概率是从原图片的起点开始到距离结尾处offset的位置处截止。
从上面可以看出来裁剪:将大图片裁剪成小的图片。下面就是将原图片的size进行扩大
3)resize_label_image_with_pad 填充图像,将图片的尺寸放大。初始化一张大小为新size的全是0的图像。然后在一定范围内随机一个填充位置,将图片放进去。除了图像放进去的位置其余位置都是0。
4)resize_label_image 扩大图像的方法直接利用scipy.misc.imresize函数,用里面的size参数设置为固定大小进行放缩。注意该函数得到的是归一化0-255范围内的数值了,如果呀归一化0-1,最后要除以255的操作。对原图的插值方式大多数选择默认的双线性插值或者是cubic插值,对于标签图片从代码来看用的插值都是‘nearest’最近邻插值方式。对标签图片进行了0-1的归一化即除以255.
5)random_resize.放缩图像,使用的是方法也是上面的函数,相同地,对标签图片也是用最近邻插值方式,对标签图片进行了0-1的归一化即除以255。只是这里size=factor是一个浮点数,并且该数是一个随机数factor=random.normalvariate(1,sig),同时要满足lower_size<=factor<=upper_size.。
random.normalvariate(1,sig) 是一个正态分布函数,以1为平均值,sig为方差。返回值原理上是负无穷到正无穷,但是由于概率的大小问题,大概率的取值平均值附近的的值,当sig越小的时候每次随机取得值越贴近均值,当sig越大的时候,随机的值波动比较大,就会远离1,但还是1附近。
6) _read_processed_image. 对图像的brightness(亮度)、contrast(对比度)、hue(色相)、saturation(饱和度)、per_image_standardization(标准化)随机化处理
7) _processe_image 对图像进行brightness(亮度)、contrast(对比度)、hue(色相)、saturation(饱和度)处理
4. create_queues 创建空的队列,定义了队列的格式。主要是用了tf.FIFOQueue函数创建了一个创建一个先入先出队列,该队列包含了入列(enqueue)和出列(dequeue)两个操作,dypes=[tf.float32,tf.int32]说明里面的都是这种tuple形式的。详细的可参考 , 和
5. start_enqueuing_threads 开始入列操作,往q里面填数据。先设置两个占位符,这两个组成tuple的形式入列q里面。使用前面_make_data_gen函数产生image和gtimage,又因为这个函数里面包含了yield也就是该函数是一个生成器,具有__next__方法。t=threading.Thread(target=enqueue_loop,args=(sess,enqueue_op,phase,gen),daemon=True)以及t.start()都是涉及到线程。可以参考
6. inputs 在队列中取出一个,然后进行图像处理(图像处理上面的第七个函数),返回image和label
7. main主函数。 coord = tf.train.Coordinator(),threads=tf.train.start_queue_runners(sess=sess,coord=coord),coord.request_stop(),coord.join(threads)这些语句都是线程方面的知识。我也不是很懂,也正在学习,可以参考 和
"""Load Kitti Segmentation Input-------------------------------The MIT License (MIT)Copyright (c) 2017 Marvin TeichmannDetails: https://github.com/MarvinTeichmann/KittiSeg/blob/master/LICENSE"""from __future__ import absolute_importfrom __future__ import divisionfrom __future__ import print_functionimport itertoolsimport jsonimport loggingimport sysimport randomfrom random import shuffleimport numpy as npimport scipy as scpimport scipy.miscimport tensorflow as tffrom tensorflow.python.ops import math_opsfrom tensorflow.python.training import queue_runnerfrom tensorflow.python.ops import data_flow_opsfrom tensorflow.python.framework import dtypesimport threadingimport osos.environ['CUDA_VISIBLE_DEVICES']='3'gpu_options = tf.GPUOptions(allow_growth=True)logging.basicConfig(format='%(asctime)s %(levelname)s %(message)s', level=logging.INFO, stream=sys.stdout)def _load_gt_file(hypes, data_file=None): """Take the data_file and hypes and create a generator. The generator outputs the image and the gt_image. """ base_path = os.path.realpath(os.path.dirname(data_file)) files = [line.rstrip() for line in open(data_file)] for epoche in itertools.count(): shuffle(files) for file in files: image_file, gt_image_file = file.split(" ") # space image_file = os.path.join(base_path, image_file) gt_image_file = os.path.join(base_path, gt_image_file) assert os.path.exists(image_file), \ "File does not exist: %s" % image_file assert os.path.exists(gt_image_file), \ "File does not exist: %s" % gt_image_file image = scipy.misc.imread(image_file, mode='RGB') # Please update Scipy, if mode='RGB' is not avaible gt_image = scp.misc.imread(gt_image_file, mode='RGB') yield image, gt_imagedef _make_data_gen(hypes, phase, data_dir): """Return a data generator that outputs image samples. @ Returns image: integer array of shape [height, width, 3]. Representing RGB value of each pixel. gt_image: boolean array of shape [height, width, num_classes]. Set `gt_image[i,j,k] == 1` if and only if pixel i,j is assigned class k. `gt_image[i,j,k] == 0` otherwise. [Alternativly make gt_image[i,j,*] a valid propability distribution.] """ if phase == 'train': data_file = hypes['data']["train_file"] elif phase == 'val': data_file = hypes['data']["val_file"] else: assert False, "Unknown Phase %s" % phase data_file = os.path.join(data_dir, data_file) road_color = np.array(hypes['data']['road_color']) background_color = np.array(hypes['data']['background_color']) data = _load_gt_file(hypes, data_file) for image, gt_image in data: gt_bg = np.all(gt_image == background_color, axis=2) gt_road = np.all(gt_image == road_color, axis=2) assert(gt_road.shape == gt_bg.shape) shape = gt_bg.shape gt_bg = gt_bg.reshape(shape[0], shape[1], 1) gt_road = gt_road.reshape(shape[0], shape[1], 1) gt_image = np.concatenate((gt_bg, gt_road), axis=2) if phase == 'val': yield image, gt_image elif phase == 'train': yield jitter_input(hypes, image, gt_image) yield jitter_input(hypes, np.fliplr(image), np.fliplr(gt_image))def jitter_input(hypes, image, gt_image): jitter = hypes['jitter'] res_chance = jitter['res_chance'] crop_chance = jitter['crop_chance'] if jitter['random_resize'] and res_chance > random.random(): lower_size = jitter['lower_size'] upper_size = jitter['upper_size'] sig = jitter['sig'] image, gt_image = random_resize(image, gt_image, lower_size, upper_size, sig) image, gt_image = crop_to_size(hypes, image, gt_image) if jitter['random_crop'] and crop_chance > random.random(): max_crop = jitter['max_crop'] crop_chance = jitter['crop_chance'] image, gt_image = random_crop_soft(image, gt_image, max_crop) if jitter['reseize_image']: image_height = jitter['image_height'] image_width = jitter['image_width'] image, gt_image = resize_label_image(image, gt_image, image_height, image_width) if jitter['crop_patch']: patch_height = jitter['patch_height'] patch_width = jitter['patch_width'] image, gt_image = random_crop(image, gt_image, patch_height, patch_width) assert(image.shape[:-1] == gt_image.shape[:-1]) return image, gt_imagedef random_crop(image, gt_image, height, width): old_width = image.shape[1] old_height = image.shape[0] assert(old_width >= width) assert(old_height >= height) max_x = max(old_height-height, 0) max_y = max(old_width-width, 0) offset_x = random.randint(0, max_x) offset_y = random.randint(0, max_y) image = image[offset_x:offset_x+height, offset_y:offset_y+width] gt_image = gt_image[offset_x:offset_x+height, offset_y:offset_y+width] assert(image.shape[0] == height) assert(image.shape[1] == width) return image, gt_imagedef random_crop_soft(image, gt_image, max_crop): offset_x = random.randint(1, max_crop) offset_y = random.randint(1, max_crop) if random.random() > 0.5: image = image[offset_x:, offset_y:, :] gt_image = gt_image[offset_x:, offset_y:, :] else: image = image[:-offset_x, :-offset_y, :] gt_image = gt_image[:-offset_x, :-offset_y, :] return image, gt_imagedef resize_label_image_with_pad(image, label, image_height, image_width): shape = image.shape assert(image_height >= shape[0]) assert(image_width >= shape[1]) pad_height = image_height - shape[0] pad_width = image_width - shape[1] offset_x = random.randint(0, pad_height) offset_y = random.randint(0, pad_width) new_image = np.zeros([image_height, image_width, 3]) new_image[offset_x:offset_x+shape[0], offset_y:offset_y+shape[1]] = image new_label = np.zeros([image_height, image_width, 2]) new_label[offset_x:offset_x+shape[0], offset_y:offset_y+shape[1]] = label return new_image, new_labeldef resize_label_image(image, gt_image, image_height, image_width): image = scipy.misc.imresize(image, size=(image_height, image_width), interp='cubic') shape = gt_image.shape gt_zero = np.zeros([shape[0], shape[1], 1]) gt_image = np.concatenate((gt_image, gt_zero), axis=2) gt_image = scipy.misc.imresize(gt_image, size=(image_height, image_width), interp='nearest') gt_image = gt_image[:, :, 0:2]/255 return image, gt_imagedef random_resize(image, gt_image, lower_size, upper_size, sig): factor = random.normalvariate(1, sig) if factor < lower_size: factor = lower_size if factor > upper_size: factor = upper_size image = scipy.misc.imresize(image, factor) shape = gt_image.shape gt_zero = np.zeros([shape[0], shape[1], 1]) gt_image = np.concatenate((gt_image, gt_zero), axis=2) gt_image = scipy.misc.imresize(gt_image, factor, interp='nearest') gt_image = gt_image[:, :, 0:2]/255 return image, gt_imagedef crop_to_size(hypes, image, gt_image): new_width = image.shape[1] new_height = image.shape[0] width = hypes['arch']['image_width'] height = hypes['arch']['image_height'] if new_width > width: max_x = max(new_height-height, 0) max_y = new_width-width offset_x = random.randint(0, max_x) offset_y = random.randint(0, max_y) image = image[offset_x:offset_x+height, offset_y:offset_y+width] gt_image = gt_image[offset_x:offset_x+height, offset_y:offset_y+width] return image, gt_imagedef create_queues(hypes, phase): """Create Queues.""" dtypes = [tf.float32, tf.int32] shape_known = hypes['jitter']['reseize_image'] \ or hypes['jitter']['crop_patch'] if shape_known: if hypes['jitter']['crop_patch']: height = hypes['jitter']['patch_height'] width = hypes['jitter']['patch_width'] else: height = hypes['jitter']['image_height'] width = hypes['jitter']['image_width'] channel = hypes['arch']['num_channels'] num_classes = hypes['arch']['num_classes'] shapes = [[height, width, channel], [height, width, num_classes]] else: shapes = None capacity = 50 q = tf.FIFOQueue(capacity=50, dtypes=dtypes, shapes=shapes) tf.summary.scalar("queue/%s/fraction_of_%d_full" % (q.name + "_" + phase, capacity), math_ops.cast(q.size(), tf.float32) * (1. / capacity)) return qdef start_enqueuing_threads(hypes, q, phase, sess): """Start enqueuing threads.""" image_pl = tf.placeholder(tf.float32) label_pl = tf.placeholder(tf.int32) data_dir = "../DATA" def make_feed(data): image, label = data return {image_pl: image, label_pl: label} def enqueue_loop(sess, enqueue_op, phase, gen): # infinity loop enqueueing data for d in gen: sess.run(enqueue_op, feed_dict=make_feed(d)) enqueue_op = q[phase].enqueue((image_pl, label_pl)) gen = _make_data_gen(hypes, phase, data_dir) gen.__next__() # sess.run(enqueue_op, feed_dict=make_feed(data)) if phase == 'val': num_threads = 1 else: num_threads = 1 for i in range(num_threads): t = threading.Thread(target=enqueue_loop, args=(sess, enqueue_op, phase, gen)) t.daemon = True t.start()def _read_processed_image(hypes, q, phase): image, label = q.dequeue() jitter = hypes['jitter'] if phase == 'train': # Because these operations are not commutative, consider randomizing # randomize the order their operation. augment_level = jitter['augment_level'] if augment_level > 0: image = tf.image.random_brightness(image, max_delta=30) image = tf.image.random_contrast(image, lower=0.75, upper=1.25) if augment_level > 1: image = tf.image.random_hue(image, max_delta=0.15) image = tf.image.random_saturation(image, lower=0.5, upper=1.6) if 'whitening' not in hypes['arch'] or \ hypes['arch']['whitening']: image = tf.image.per_image_whitening(image) logging.info('Whitening is enabled.') else: logging.info('Whitening is disabled.') image = tf.expand_dims(image, 0) label = tf.expand_dims(label, 0) return image, labeldef _dtypes(tensor_list_list): all_types = [[t.dtype for t in tl] for tl in tensor_list_list] types = all_types[0] for other_types in all_types[1:]: if other_types != types: raise TypeError("Expected types to be consistent: %s vs. %s." % (", ".join(x.name for x in types), ", ".join(x.name for x in other_types))) return typesdef _enqueue_join(queue, tensor_list_list): enqueue_ops = [queue.enqueue(tl) for tl in tensor_list_list] queue_runner.add_queue_runner(queue_runner.QueueRunner(queue, enqueue_ops))def shuffle_join(tensor_list_list, capacity, min_ad, phase): name = 'shuffel_input' types = _dtypes(tensor_list_list) queue = data_flow_ops.RandomShuffleQueue( capacity=capacity, min_after_dequeue=min_ad, dtypes=types) # Build enque Operations _enqueue_join(queue, tensor_list_list) full = (math_ops.cast(math_ops.maximum(0, queue.size() - min_ad), dtypes.float32) * (1. / (capacity - min_ad))) # Note that name contains a '/' at the end so we intentionally do not place # a '/' after %s below. summary_name = ( "queue/%s/fraction_over_%d_of_%d_full" % (name + '_' + phase, min_ad, capacity - min_ad)) tf.summary.scalar(summary_name, full) dequeued = queue.dequeue(name='shuffel_deqeue') # dequeued = _deserialize_sparse_tensors(dequeued, sparse_info) return dequeueddef _processe_image(hypes, image): # Because these operations are not commutative, consider randomizing # randomize the order their operation. augment_level = hypes['jitter']['augment_level'] if augment_level > 0: image = tf.image.random_brightness(image, max_delta=30) image = tf.image.random_contrast(image, lower=0.75, upper=1.25) if augment_level > 1: image = tf.image.random_hue(image, max_delta=0.15) image = tf.image.random_saturation(image, lower=0.5, upper=1.6) return imagedef inputs(hypes, q, phase): """Generate Inputs images.""" if phase == 'val': image, label = q[phase].dequeue() image = tf.expand_dims(image, 0) label = tf.expand_dims(label, 0) return image, label shape_known = hypes['jitter']['reseize_image'] \ or hypes['jitter']['crop_patch'] if not shape_known: image, label = q[phase].dequeue() nc = hypes["arch"]["num_classes"] label.set_shape([None, None, nc]) image.set_shape([None, None, 3]) image = tf.expand_dims(image, 0) label = tf.expand_dims(label, 0) if hypes['solver']['batch_size'] > 1: logging.error("Using a batch_size of {} with unknown shape." .format(hypes['solver']['batch_size'])) logging.error("Set batch_size to 1 or use `reseize_image` " "or `crop_patch` to obtain a defined shape") raise ValueError else: image, label = q[phase].dequeue_many(hypes['solver']['batch_size']) image = _processe_image(hypes, image) # Display the training images in the visualizer. tensor_name = image.op.name tf.summary.image(tensor_name + '/image', image) road = tf.expand_dims(tf.to_float(label[:, :, :, 0]), 3) tf.summary.image(tensor_name + '/gt_image', road) return image, labeldef main(): """main.""" with open('../hypes/KittiSeg.json', 'r') as f: hypes = json.load(f) q = {} q['train'] = create_queues(hypes, 'train') q['val'] = create_queues(hypes, 'val') data_dir = "../DATA" # _make_data_gen(hypes, 'train', data_dir) image_batch, label_batch = inputs(hypes, q, 'train') logging.info("Start running") with tf.Session(config=tf.ConfigProto(gpu_options=gpu_options)) as sess: # Run the Op to initialize the variables. init = tf.initialize_all_variables() sess.run(init) coord = tf.train.Coordinator() start_enqueuing_threads(hypes, q, 'train', sess) logging.info("Start running") threads = tf.train.start_queue_runners(sess=sess, coord=coord) for i in itertools.count(): image = image_batch.eval() gt = label_batch.eval() scp.misc.imshow(image[0]) gt_bg = gt[0, :, :, 0] gt_road = gt[0, :, :, 1] scp.misc.imshow(gt_bg) scp.misc.imshow(gt_road) coord.request_stop() coord.join(threads)if __name__ == '__main__': main()
综上所述:该文件就是prepare the data。
转载地址:https://blog.csdn.net/zz2230633069/article/details/82385921 如侵犯您的版权,请留言回复原文章的地址,我们会给您删除此文章,给您带来不便请您谅解!
发表评论
最新留言
关于作者
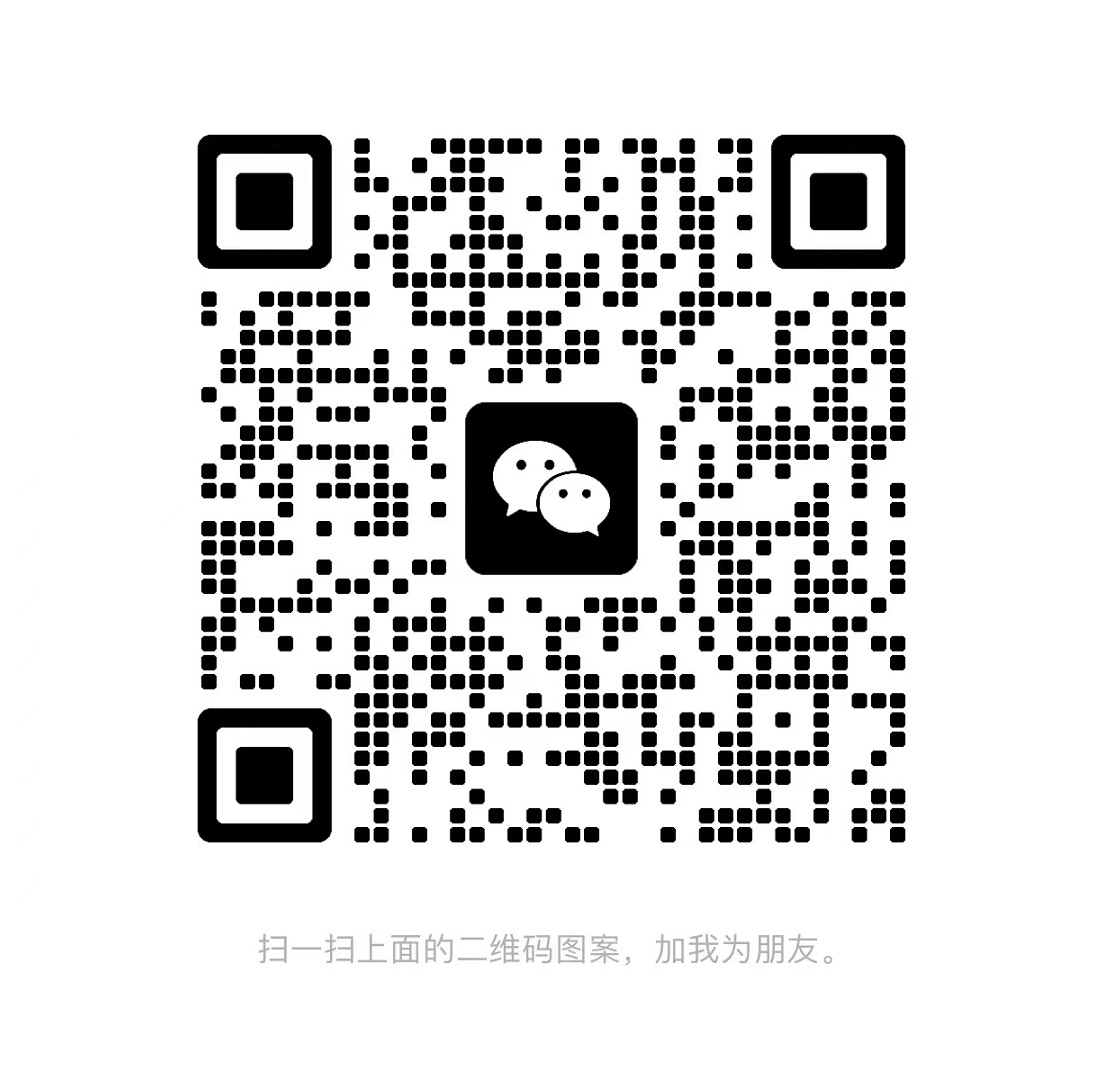