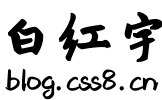
本文共 1666 字,大约阅读时间需要 5 分钟。
HiPPO: Recurrent Memory with Optimal Polynomial Projections
HiPPO is a cutting-edge recurrent neural network architecture designed to leverage optimal polynomial projections for memory modeling. This innovative approach addresses the critical challenge of capturing long-term dependencies in sequential data, which is essential for various applications ranging from natural language processing to time-series analysis.
The HiPPO model introduces a novel state space model (SSM) that effectively manages the trade-off between computational efficiency and the capacity to capture complex temporal patterns. By employing optimal polynomial projections, HiPPO achieves a balance between expressiveness and stability, making it particularly suitable for scenarios where both short-term and long-term dependencies are significant.
One of the key strengths of HiPPO lies in its ability to mitigate the vanishing gradient problem, a common issue in neural networks that can hinder the learning of long-term dependencies. This is accomplished through the strategic design of the polynomial projection mechanism, ensuring that gradients do not diminish excessively over time.
In addition to its technical prowess, HiPPO is computationally efficient, making it accessible for deployment in practical applications. The model?s optimal polynomial projections not only enhance its expressive power but also contribute to its robustness and generalization capability.
Ultimately, HiPPO represents a significant advancement in the field of neural network architectures, offering a promising solution to the challenges of sequential data processing and long-term dependency modeling.
发表评论
最新留言
关于作者
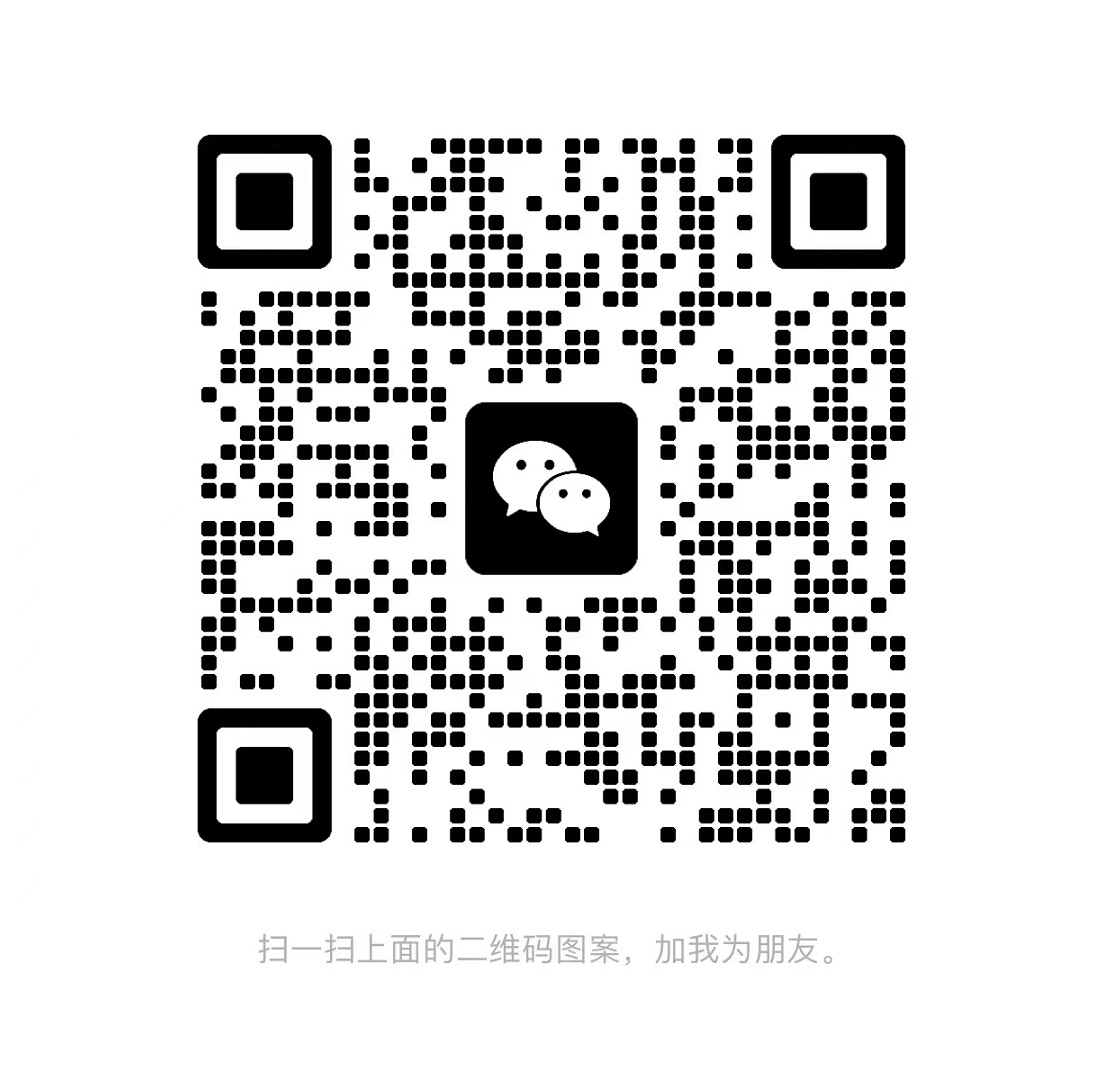